Personalized Experiences Through Advanced Algorithms
In today’s digital landscape, personalization is the cornerstone of user engagement. Whether it’s a video on YouTube, a song on Spotify, or a product on Amazon, recommendations drive user satisfaction. Behind these experiences lie recommendation engines deep learning models and fueled by humongous amounts of data. These engines are intelligent and learn from the behavior and preferences to suggest the most relevant content, creating seamless and tailored digital experiences.
Unlike traditional methods, which rely heavily on manually curated rules, guidance engines deep studying continually adapt with time to serve more competently shifting user patterns. They learn patterns, identify anomalies, and continually improve without human intervention.
The Crucial Role of Big Datasets
High-volume, high-quality data is the foundation of quality recommendation systems. Data within recommendation engines datasets include user history, browsing behavior, purchase history, ratings, and even sometimes time spent on content view at times. The higher the data within a dataset, the more accurately the engine can understand the intent of the users.
These suggestion engines data sets are preprocessed to minimize noise and normalize input to provide better accuracy. Deep learning algorithms are then trained against such data to extract fine-grained relationships otherwise not discoverable. There are millions of users contributing content every day, so the scale is both a strength and a weakness, enabling the engines to continuously hone their predictions.
For instance, Netflix employs enormous recommendation engines datasets in order to personalize recommendations for more than 200 million active users globally. Each interaction adds yet another degree of smartness to its platform.
Deep Learning Architectures Driving Innovation
There are numerous deep learning models employed in recommendation engines deep learning that are superior to one another in other ways. One of these is the Neural Collaborative Filtering (NCF) that is superior compared to the matrix factorization methods of yesteryears. Such a model employs user and item embeddings to make predictions with improved performance over sparse data.
Recurrent Neural Networks (RNNs) are applicable in sequential recommendations, for instance, where to recommend the next song a user will play. CNNs, however, are able to process visual content and be applied to recommend similar images or products.
In contemporary systems, hybrid approaches are the norm. These combine a range of architectures and sources of input e.g., user clicks, queries, and demographics—to construct high-powered guidance engines deep studying techniques. These systems don’t simply suggest what’s popular, they seek to suggest what’s relevant to an individual.
Real-Time Adaptation and Feedback Loops
Recommendation engines today are no longer fixed; they are updated continuously with real-time feedback. Each time the users engage with the platform, their usage feeds into the recommendation engines datasets, which, in turn, re-tune the deep learning models.
In this way, websites can react to changing interests in real time. If a user begins to search for garden gear, then the engine will change its suggestions to reflect that. With the more clicks and interactions, the system becomes more discriminating.
Streaming media platforms such as Spotify and online retailers such as Amazon heavily depend on these feedback loops. The combination of guidance engines deep studying with new data ensures the recommendations are always up to date, relevant, and interesting.
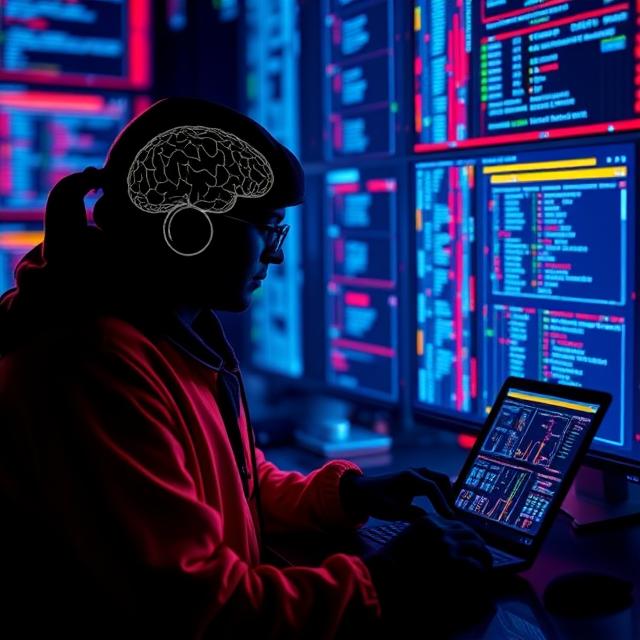
How deep learning and datasets power recommendation engines
Ethics, Bias, and Data Privacy
Although powerful, recommendation engines have ethical implications. Since recommendation engine datasets can reflect social biases, the deep models used can exacerbate this. This will lead to filter bubbles or biased content suggestions reducing user exposure to diverse information.
To address this, researchers use fairness constraints and debiasing techniques. Exploitability of recommendation engines deep learning model operation becomes more critical to attain user trust. Secondly, data privacy laws such as GDPR require platforms to explain data usage as well as offer an opt-out option.
Attaining harmony between precision and ethics is essential to have AI-powered personalization that is sustainable. With these technologies continuing to develop, ethical support and open practice must keep pace.
Business Impact and Future Potential
The business potential for recommendation engines is enormous. From monetization to user retention, business from different industries depends on these engines. Organizations can make personalized offerings in real-time using recommendation engines deep learning, which decreases churn and enhances customer loyalty.
With every advancement in computing power and introduction of new methods such as transformers and reinforcement learning, the efficiency of these engines will further increase. Future innovations can include merging recommendation engines datasets with behavioral psychology or augmented reality to provide even more personalized experiences.
Recommendation engines datasets powered by massive recommendation engines deep learning offer precise, evolving, and personalized user experiences.
The Impact of AI Video on Production Kits and Content Providers
The Future of Privacy-Focused in AI Development Life Cycle Tools